Leaked information about Qwen3 Coming Soon
Analysis of Qwen3’s technical features and open-source strategy—based on leaks from social media—helps readers understand the upcoming AI model update.
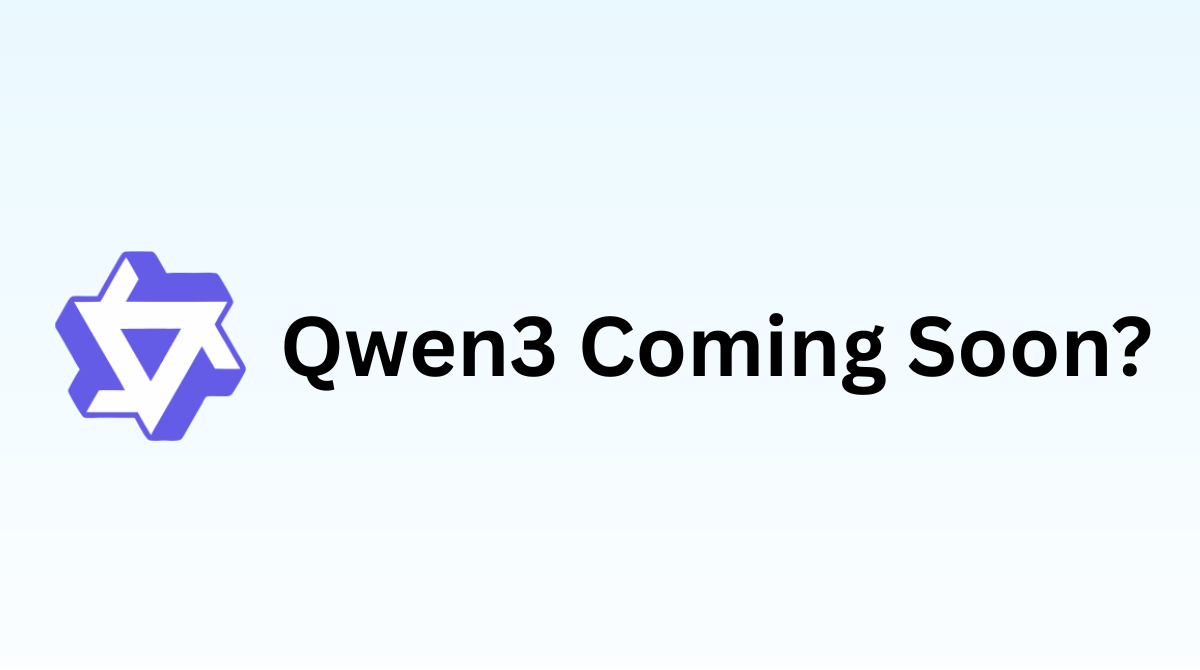
In today's fiercely competitive AI model landscape, a heavyweight contender is about to enter the arena. According to multiple social media sources, Alibaba Cloud's Qwen3 (Tongyi Qianwen 3.0) is set to be officially released soon. It is important to note that the information in this article is primarily sourced from discussions and shares on social media. Readers should maintain a cautious attitude and await the official release for accurate information.
Based on leaks from social media and the community, Qwen3 features dual Dense and MoE architecture paths, up to 238B parameters, a 32K context length, and significantly enhanced multilingual, reasoning, and coding capabilities, while maintaining an open-source friendly strategy.
As one of the representative works of large language models, the Qwen series has maintained a steady iteration rhythm. Based on information from various social media sources, this article provides a preliminary analysis of Qwen3's technical features, open-source strategy, helping readers understand this upcoming AI model update.
Technical Architecture: Innovative Dual-Path Design
According to discussions on multiple social media platforms, Qwen3 adopts a dual-path technical architecture strategy, simultaneously advancing Dense and MoE (Mixture of Experts) model structures to provide flexible options for different application scenarios.
Parameter Scale and Model Series
In terms of parameter scale, Qwen3 demonstrates a comprehensive product line layout:
- Full Spectrum Coverage: From 0.6B, 1.7B, 4B, 8B, 30B-A3B to the flagship 238B, meeting deployment needs from mobile devices to cloud servers
- MoE Architecture Advantages: MoE version design increases activated parameters several-fold, significantly enhancing model capabilities while maintaining inference efficiency
- Context Length: Maximum support for 32K context window, meeting long document processing requirements
This full-spectrum coverage strategy enables Qwen3 to simultaneously meet the lightweight requirements of edge devices and the performance requirements of high-end servers, demonstrating clear technical versatility advantages.
Training Data and Multilingual Capabilities
Qwen3's training data scale and quality have also seen significant improvements:
- Training Corpus Expansion: Based on 119+ languages, with pre-training token scale reaching 36 trillion, three times that of Qwen2.5
- Data Quality Optimization: Extensive adoption of high-quality data in STEM, programming, reasoning, and other fields, enhancing performance in professional domains
- Multilingual Support: Significantly enhanced multi-turn dialogue and translation capabilities, with notable improvements in multilingual generalization and instruction following
These improvements enable Qwen3 to demonstrate stronger capabilities when processing multilingual content and professional domain tasks, especially in bidirectional translation and understanding between Chinese and English.
Innovative Mechanisms and Architectural Optimizations
Qwen3 introduces multiple technical innovations to enhance model performance:
- qk-layernorm: Optimizes attention mechanisms, improving model stability
- Progressive Long-Text Training: Improves long text understanding capabilities
- Scaling Law Hyperparameter Tuning: Optimizes model training efficiency and effectiveness
- Dual-Mode Switching: Supports single-model "thinking" and "non-thinking" dual-mode switching, compatible with general dialogue and advanced reasoning tasks
These technical innovations enable Qwen3 to significantly improve inference stability, generalization capabilities, and reasoning precision while maintaining high performance, particularly excelling in complex reasoning tasks.
Performance Evaluation: Surpassing Previous Generations, Approaching Industry-Leading Levels
According to community evaluations and social media discussions, Qwen3 has achieved significant progress on multiple key metrics. However, this information has not been officially confirmed, and readers should maintain appropriate skepticism.
Benchmark Tests and Actual Experience
Community users widely report that Qwen3 demonstrates qualitative leaps in complex reasoning tasks:
- Natural Language Understanding: Qwen3's main models (such as Qwen3-8B) comprehensively surpass Qwen2.5 in natural language understanding tasks
- Logical Reasoning: Significant improvements in mathematical reasoning and logical analysis tasks
- Code Generation: Excellent performance in code scenarios, approaching industry-leading levels
- Context Utilization: 32K context length performs stably in practical applications, though still has some distance compared to the 128K context of some competitors
Particularly in problems requiring multi-step thinking, the model demonstrates stronger coherence and accuracy, which is significant for building complex AI applications.
Open-Source Strategy and Ecosystem Building: Low Barriers Promoting Widespread Application
The Qwen series has always been known for its open-source friendliness, and Qwen3 continues and strengthens this strategy.
Open-Source and Commercial Policies
Qwen3's open-source strategy has significantly promoted its ecosystem development:
- Full Open-Source: Qwen3's full weights are available for download on platforms like ModelScope and Hugging Face
- Free Basic Inference: Free basic inference services lower the usage threshold
- Community Activity: Developer community forks have exceeded one hundred thousand, with an active ecosystem
- Business Model: Plans to subsequently release enterprise-level customization, API, and cloud value-added services, maintaining a low-barrier/high-compatibility commercial strategy
This open-source strategy enables more upstream and downstream enterprises and developers to incubate innovative products with Qwen as the foundation, forming a virtuous ecosystem cycle.
References
[1] @kimmonismus. (2025, April 21). "Qwen3 parameter table and architecture analysis" [Tweet]. X.com. https://x.com/kimmonismus/status/1916818352485413038
[2] @karminski3. (2025, April 21) . "Qwen3 MoE architecture details and screenshots" [Tweet]. X.com. https://x.com/karminski3/status/1916835446400958936
[3] u/techexplorer. (2025, April 20) . "Qwen3 technical elements analysis" [Online forum post]. Reddit. https://www.reddit.com/r/LocalLLaMA/comments/1k9qxbl/comment/mpgfxul/
[4] u/tjuene. (2025, April 20) . "Qwen3 context length comparison with Gemma3" [Online forum post]. Reddit. https://www.reddit.com/r/LocalLLaMA/comments/1k9qxbl/comment/mpgqpcr/
[5] @MarkusOdenthal. (2025, April 21) . "Qwen3 local running experience" [Tweet]. X.com. https://x.com/MarkusOdenthal/status/1916832194561003773
[6] Qwen Official. (2025) . "Qwen model repository". ModelScope. https://modelscope.cn/organization/Qwen
[7] Qwen Official. (2025) . "Qwen model repository". Hugging Face. https://huggingface.co/Qwen
Note: This article is compiled based on publicly available information from social media. Readers should maintain a cautious attitude. Specific details are subject to official release by Alibaba Cloud.